Test data¶
Aim¶
Provide a test dataset that has a diurnal cycle plus a little noise. These data are used for testing the visualisation and 24 hour predictions.
Summary¶
The Flask API handles the creation of these data when running app.py. Make sure to drop and recreate, or truncate all rows from the home_sensor_data table. If the table is empty then 300 records are added to the database with historical datestamps at 1 hour intervals starting from the current date and time.
Note
The following code blocks are in separate files. The method for creating data is in predictions.py which lives next to app.py.
Creating data¶
def createData(startDate, nHourInterval, nRecords):
"""
Creates a list of dictionaries containing timeseries data points.
This function walks backwards from the startDate providing values
at each interval (nHourInterval) for as many records (nRecords)
as required.
"""
output = []
for n in range(nRecords):
# vars
dt = startDate - timedelta(hours=(n * nHourInterval))
ds = dt.isoformat()
hr = dt.hour
rn = random.random()
if (hr < 12):
val = round((10 + (hr % 12)) + rn, 3)
else:
val = round((10 - (hr % 12)) + 12 + rn, 3)
d = {"name": "TestData", "location": "Home", "category": "actual",
"measurementType": "temp", "value": val, "dsCollected": ds}
# use insert instead of append to put the
# record at the start of the list
output.insert(0, d)
return output
Testing¶
import pytz
from datetime import *
import predictions
import matplotlib.pyplot as plt
import dateutil.parser as parser
# variables
STARTDATE = datetime.today().replace(
tzinfo=pytz.timezone('Australia/Sydney'))
NHOURINTERVAL = 1
NRECORDS = 300
# create data
pcd = predictions.createData(STARTDATE, NHOURINTERVAL, NRECORDS)
# Format example
# [{
# 'name': 'TestData',
# 'location': 'Home',
# 'dsCollected': '2017-11-25T08:18:20.167450+10:05',
# 'category': 'actual',
# 'value': 18.947,
# 'measurementType': 'temp'
# }]
# reorganise data for plotting
data = {"ds":[], "val":[]}
# loop array of dictionaries
for elem in pcd:
# inspect dictionary
for key, value in elem.items():
# handle keys of interest
if key == 'dsCollected':
# Parse dates from string to datetime format
data["ds"].append(parser.parse(value))
elif key == "value":
data["val"].append(value)
else:
continue
plt.figure(figsize=(12,4))
plt.plot(data["ds"], data["val"])
plt.show()
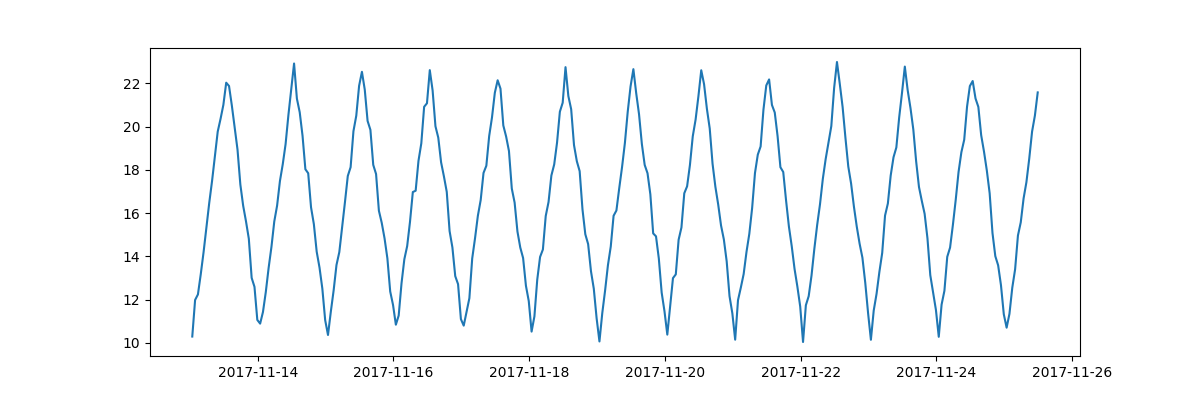
Flask implementation¶
# REMINDER - deleting records
# DROP TABLE home_sensor_data;
# CREATE TABLE home_sensor_data;
sendat = HomeSensorData.query.all()
createFakeData = True
if createFakeData and len(sendat) == 0:
STARTDATE = datetime.today().replace(tzinfo=pytz.timezone('Australia/Sydney'))
NRECORDS = 300
NHOURINTERVAL = 1
td = predictions.createData(STARTDATE, NHOURINTERVAL, NRECORDS)
for record in td:
sendat = HomeSensorData(
name = record['name'],
location = record['location'],
category = record['category'],
measurementType = record['measurementType'],
value = record['value'],
dsCollected = record['dsCollected']
)
db.session.add(sendat)
db.session.commit()